Air Force researchers ask industry for SWaP-constrained embedded computing for artificial intelligence (AI)
ROME, N.Y. – U.S. Air Force researchers are asking for industry help in making big improvements in small, lightweight embedded computing for artificial intelligence (AI) and machine learning (AI/ML) capabilities in an embedded computing environment.
Officials of the Air Force Research Laboratory's Information Directorate in Rome, N.Y., issued a broad agency announcement on Thursday (FA875019S7007) for the Robust and Efficient Computing Architectures, Algorithms, and Applications For Embedded Deep Learning.
The potential $99 million five-year project seeks to achieve orders of magnitude improvement in size, weight and power (SWaP) for deploying artificial intelligence and machine learning capabilities in an embedded computing environment.
Researchers are asking industry for embedded computing technologies with improved autonomy, intelligence, and assurance for command, control, communications, computers, intelligence, surveillance and reconnaissance (C4ISR) applications in SWaP-constrained Air Force applications. Of particular interest are advanced efficient computing architectures, algorithms, and applications.
Related: AI, machine learning driving embedded bus-and-board development
The Air Force needs unconventional computing architectures for pattern recognition, event reasoning, decision making, adaptive learning, and autonomous tasking on energy-efficient Air Force manned and unmanned aircraft.
A major focus area is neuromorphic computing -- or brain-inspired computing that involves processors more advanced than more-traditional the Von Neumann architectures. This kind of design could lead to unconventional circuits based on emerging nanotechnology like memristors and nano-photonics, researchers say.
There is a need for in-hardware and efficient adaptive learning architectures and algorithms, as well as novel artificial intelligence and machine learning algorithms optimized for neuromorphic computing hardware architectures.
Air Force researchers want to demonstrate modular computing for future real-time embedded plug-and-play capabilities. Technologies could include artificial intelligence and machine learning models for big data analytics for sensor processing; data fusion algorithms for situational awareness and sense making; and autonomous decision making.
Modular designs should support interchangeable sensors, with automatic software reconfiguration based on available resources. Companies participating should choose computing and interfaces to accommodate expected growth in data bandwidth in future systems. SWaP optimization is a priority.
Other considerations are ways to validate and improve scalability and security for artificial intelligence and machine learning technologies; deep-learning models and algorithms; and power-aware and energy-optimized deep learning models and algorithms for embedded computing.
Companies interested should email white papers no later than 27 May 2022 to the Air Force's Albert Frantz at [email protected]. Other deadlines for white paper submissions are 30 April and 31 July 2019; 29 May 2020; and 28 May 2021.
Those submitting promising white papers will be asked to submit full proposals. Email technical questions or concerns to Albert Frantz at [email protected], and contracting questions to Amber Buckley at [email protected].
More information is online at https://beta.sam.gov/opp/710000c429424dbf87606d0bc569d29e/view.
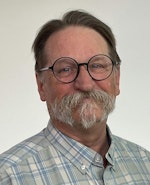
John Keller | Editor-in-Chief
John Keller is the Editor-in-Chief, Military & Aerospace Electronics Magazine--provides extensive coverage and analysis of enabling electronics and optoelectronic technologies in military, space and commercial aviation applications. John has been a member of the Military & Aerospace Electronics staff since 1989 and chief editor since 1995.