Artificial intelligence (AI) takes its place in sensor, signal, and image processing
Military threats are accelerating at machine speed, so military forces are adding artificial intelligence (AI) and machine learning to their arsenals of sensor, signal, and image processing to analyze vast streams of data in real time. By pushing computing power to the tactical edge in aircraft, armored vehicles, and even soldier-deployed systems, AI-driven systems minimize decision-making delays and enhance situational awareness. Coupled with distributed processing architectures, these technologies allow for autonomous platforms and crewed-uncrewed teams to act with greater speed, flexibility, and resilience in contested environments.
Adopting AI-driven sensor, signal, and image processing at the edge enhances military operations by reducing reliance on centralized command hubs and minimizing the effects of network latency or signal interference in contested environments. By decentralizing computational workloads across interconnected platforms, forces can analyze and respond to critical intelligence at the source -- whether it's an uncrewed aerial system (UAS) detecting threats in real-time or a ground vehicle processing electronic warfare (EW) signals on the move. As defense organizations embrace these advancements, they speed autonomous decision-making, and strengthen mission effectiveness across land, sea, air, space, and cyber domains.
"There is a trend towards consolidating sensor processing onto a single platform often sharing common processing resources with an open standard architecture sub-system. This drives needs in SOSA aligned hardware for architecture that allows GPGPU plug-in cards to be mated with SBC plug-in cards, while supporting payload slots that may require RF payloads in the same system," says Mark Littlefield, director of system products at Elma Electronic in Fremont, Calif. Littlefield is active contributor to several VITA and Sensor Open Systems Architecture (SOSA) technical working groups.
AI-Driven Signal Processing and Sensor Fusion for Situational Awareness
Rodger Hosking, director of sales at Mercury Systems in Andover, Mass., explains that advanced signal processing aims to transform data into situational awareness through surveillance, targeting, and autonomous systems.
"New sensor initiatives include hyperspectral and multispectral imaging to gain information beyond human vision. Quantum sensors using ultra-sensitive gyroscopes, magnetometers, and accelerometers support GPS-denied navigation and submarine detection, and AI-enabled radar and LiDAR using machine learning algorithms improve target detection, tracking, and clutter reduction," Hosking says. "New signal processing strategies use AI and machine learning for deep learning-based signal analysis to automate EW threat identification and RF spectrum management. Cognitive EW enhances adaptive jamming and electronic countermeasures to counter enemy signals in real time. Neural network-based noise reduction enhances signal clarity in high-interference environments."
Mercury's Hosking explains that advanced image processing for developments in intelligence, surveillance, and reconnaissance (ISR) technologies includes AI-powered synthetic aperture radar (SAR) image analysis for all-weather, day-and-night surveillance, along with real-time object recognition.
"Deep learning models rapidly detect, classify, and track objects from satellite and drone imagery. Edge AI is being used to reduce reliance on cloud-based computation, allowing real-time image analysis on UAVs and satellites," Hosking says. "New sensor fusion initiatives include cross-domain data fusion to integrate radar, IR, EO, sonar, and SIGINT data for a comprehensive battlefield picture. Distributed sensing networks help swarm UAVs and smart sensor grids share real-time data for collaborative targeting. Automated anomaly detection exploits AI-assisted correlation of sensor feeds to detect hidden threats, like stealth aircraft and cyber intrusions."
Sensor Fusion and Edge AI Tackle Data Challenges in Defense Systems
Brian Russell, director of mil-aero solutions at Aitech in Chatsworth, Calif., explains that the trends of bringing robust capabilities to the battlefield and embracing uncrewed platforms of all sizes are evident. "is a there is an increasing need for smaller and lighter sensor packages as well as multi-sensor fusion of that data via edge computing. In addition, the use of robust AI/machine learning algorithms to reduce both operator workloads and the amount of data moving across the battlefield is just as critical to data processing in today’s military and aerospace applications."
David Tetley, director of embedded computing at Elma Electronic, says combining data into actionable intelligence from seemingly unrelated technologies, such as electro-optical (EO) and infrared (IR), presents an initial challenge.
"The first challenge is data ingest," Tetley says. "Different sensor types have very different digitization front ends and convey the data using different protocols and electrical standards. For example, EO/IR data from a camera gimbal may present its data on a 3G-SDI interface, whilst RF data may be transmitted as IQ data over a high-speed network or over PCIe from a RF data acquisition board. Therefore, system architectures need flexibility to accommodate different sensor interface types and data bandwidths.
He continues, "As different sensors have different latencies, there is also the challenge to register and align the data from the different sensor entities in the time domain. This is facilitated by accurate timestamps via global clocking mechanisms and use of IEE802.1AS."
Denis Smetana, senior product manager at Curtiss-Wright Defense Solutions in Ashburn, Va., says "Any time you are trying to combine data from different sources you need to normalize the data so that sampling rates, resolution, data characteristics, etc. can be combined together in meaningful ways. Otherwise, a difference in the definition of the data can cause distorted results."
Mercury's Hosking notes that as sensors operate at different frequencies, resolutions, and bandwidth and produce diverse data formats and sampling rates in addition to different military platforms in coalition forces, the resultant data can result in costly errors.
"Time delays between different sensors can cause misalignment in data fusion, and out-of-sync timestamps can lead to incorrect object tracking or misinterpretation of threats," Hosking says. "Bandwidth constraints may prevent real-time data transmission from distributed sensors. Noisy or incomplete data from one sensor may mislead fusion algorithms, and sensor biases, drifts, or environmental interferences need compensation."
He continues, "Multiple sensors may provide contradictory data, and false alarms from one sensor can bias the entire fusion system. Accurate object association is difficult when tracking several entities across sensors with different fields of view."
While modern systems enable unprecedented situational awareness, they also produce vast amounts of data. This leads to increased power consumption. Justin Moll, vice president of sales and marketing for Pixus Technologies in Waterloo, Ontario, states that these trends will necessitate the development of new architectures. "AI and machine learning are generating unprecedented capabilities for advanced signal processing and data crunching. The drawback from a chassis perspective is these powerful chipsets are driving up the wattage of the PICs [plug-in cards]. The extreme high speeds and performance requirements of these systems will start to drive the market to a new generation of architecture, such as VITA 100."
How AI and Machine Learning Power Signal Intelligence and Electronic Warfare
According to Ken Grob, director of embedded technologies at Elma Electronic, AI is providing a helping hand in sifting through the mountain of data that today's sensor technologies provide. "Bringing in AI processing into a sensor can assist in processing the data stream. In image sensors, data streams can be preprocessed at the sensor. In the case of RF systems signals can be analyzed through the use AI processing. Further, in a communication example, voice comms can be converted from language to language, and processed for keywords of phrases. Outputs can be audio or text."
Aitech's Russell says that AI and machine learning has enabled processing more data at the edge and send only the pertinent data packets and information to centralized computing and operators. "An example would be target recognition using AI/machine learning via a sensor," Russell says. "Instead of sending data and image for every target in the field of view, the user could select basic 'only send defined threats' data, hence reducing the required bandwidth of data flow and also reducing operator workload and latency. The operator would only have to process and react to true threats."
Russell remarks that Aitech's A230 Vortex is a standout as a rugged edge compute system ideal for AI at the edge and distributed systems and is available with the NVIDIA Jetson AGX Orin Industrial System-on-Module. "Its Ampere GPU features up to 2048 CUDA cores and 64 Tensor cores, delivering up to 248 TOPS and ensuring remarkable energy efficiency for AI-based local processing right alongside your sensors," Russell says. "In addition, the system includes two dedicated NVIDIA Deep-Learning Accelerator (NVDLA) engines, tailored for deep learning applications. With its compact size, the A230 Vortex sets the standard as the most advanced solution for AI, deep learning, and video and signal processing in next generation autonomous vehicles, surveillance and targeting systems, EW systems, and more."
Hosking at Mercury Systems says that in addition to target identification with EO/IR, SAR, and radar imagery, "Cognitive radar systems dynamically adjust waveforms based on environmental conditions and threats. AI improves clutter suppression, reducing false alarms in maritime and airborne surveillance. machine learning-based electronic warfare (EW) threat classification enables real-time signal identification and jamming. Bayesian networks and deep learning improve sensor fusion for more accurate tracking of fast-moving threats, and AI-driven data association algorithms resolve conflicting sensor inputs and enhance object correlation."
Mercury's DRF2270 System-on-Module (SoM) and DRF5270 3U board are the latest additions to its direct RF digital signal processing product line, using Altera FPGAs to analyze data across a broad range of the electromagnetic spectrum. The DRF2270 is an eight-channel SoM capable of converting analog and digital signals at a rate of 64 gigasamples per second.
The DRF5270 integrates the DRF2270 SoM into a 3U defense-ready board, featuring 10, 40, and 100 Gigabit Ethernet optical interfaces. The modular design of the SoM allows for customization to specific applications without requiring a full board redesign. Additionally, the DRF2270 can be incorporated into other small-form-factor or custom configurations.
Why Defense Systems Are Pushing for Faster Data Speeds and Smarter Edge Processing
Bob Vampola, vice president of aerospace and defense business at Microchip Technology in Chandler, Ariz., identified a quartet of trends driving the development needs of today's signal processing technologies. First, Vampola says there is a need for more resolution at a higher number of frames per second.
"One consequence is higher bus speeds to accommodate the increase in data," Microchip's Vampola says. "The second is high-speed Ethernet for the data bus. This trend isn’t new, however, it is gaining speed and broader acceptance. The third, for many imaging applications, constraints like available power, power dissipation and available volume drive a non-GPU approach. The trade-off is around performance (typically speed) vs size and power budgets. And lastly, image processing at the edge is gaining traction. In this case, edge means placing a dedicated processor near the camera, extracting meaning from the image data there, and transmitting the meaning rather than the entire image data to a more central node. The result is lower bus speeds and relaxed thermal management concerns.
"As compute workloads move to the edge, [Microchip's] PolarFire SoC and PolarFire FPGAs offer 30–50% lower total power than competing mid-range FPGAs, with five to ten times lower static power, making them ideal for a new range of compute-intensive edge devices, including those deployed in thermally and power-constrained environments," Vampola continues. "PolarFire SoC and PolarFire FPGA Smart Embedded Vision solutions include video, imaging and machine learning IP and tools for accelerating designs that require high performance in low-power, small form factors across the industrial, medical, broadcast, automotive, aerospace and defense markets."
Curtiss-Wrights' Smetana also identified four sensor processing trends in mil-aero systems, including the necessity to manage a large volume of high-resolution data, resulting in a demand for increased bandwidth capacity. "In order to accommodate the higher bandwidth there is a stronger push for 100 Gigabit Ethernet fabrics, Gen4 or even Gen5 PCIe links, and an acceleration in smart sensors with fiber optic connections to FPGAs," says Smetana, acknowledging a growing demand for several intelligence (multi-INT) capabilities and sensor fusion and the necessity of incorporating GPUs to assist with the required processing volume.
"Although this has to be balanced with the power and heat they generate and challenges related to securing GPU data," notes Smetana. "For larger systems where 6U VPX cards are used, we are seeing a significant increase in the need for Liquid Flow Through (LFT) cooling as it is the only way to cool the higher-end processing devices whether they are general purpose processors, FPGAs, or GPUs."
Curtiss-Wright Defense Solutions' CHAMP-FX7 is a rugged real-time processing board featuring AMD’s Versal Adaptive SoC devices. These Adaptive SoCs integrate FPGA programmable logic with Arm-based processing and high-performance I/O, supporting floating-point and integer arithmetic for signal processing and AI applications.
The board is designed for high-speed data processing, with over 100 transceivers supporting 100-Gigabit Ethernet, PCIe Gen4, and other high-rate protocols over VPX backplanes. It includes hard IP blocks for DDR4 SDRAM, PCIe, and Ethernet, with a low-latency Programmable Network-on-Chip for efficient data movement.
The CHAMP-FX7 incorporates two VP1702 Versal Premium devices on a 6U SOSA-aligned VPX platform. It features quad 100-Gigabit Ethernet ports and 32-lane PCIe Gen4 connectivity, enabling high-speed data transfer between SoCs, processors, GPUs, and network switches within embedded VPX systems.
VITA 100 and SOSA: What’s Ahead for High-Speed Defense Computing
Elma Electronic's Littlefield explains that the forthcoming VITA 100 standard will play a large role starting in 2026. "Elma is not only participating in the crafting of the VITA 100 standard, but it is taking steps to ensure that we can create and manufacture VITA 100 products that perform to expectations," says Littlefield. "It’s not simply a matter of sticking the new connectors on the board, it requires careful consideration of signal integrity issues, the proper choice of materials and route paths and dimensions, and an infrastructure to test and validate that performance targets -- with proper margins -- are being met. The performance increases that VITA 100 will bring will be critical for next-generation signal and image processing platforms.
He continues, "Elma is introducing its 'AI-Ready' SOSA aligned backplanes that support a x16 Gen 4 PCIe interface on the Expansion Plane between a Computer Intensive SBC and NVIDIA GPU, thus maximizing sensor data bandwidth and minimizing latency. This is coupled with support of 100-Gigabit Ethernet on the data plane to support the wide bandwidth need for VITA 49 RF data, as required by MORA and SOSA, to convey data between the front-end and back-end RF processing entities."
Pixus Technologies also has its eyes on the coming VITA 100. Moll remarks that "Pixus has developed several 3U OpenVPX ATR enclosure designs for SOSA requirements. This includes incorporating fiber and optical interfaces through the backplane, advanced cooling over fins for the hotter modules, and speeds of 100-Gigabit Ethernet and beyond.
He continues, "Pixus has also designed a SOSA aligned backplane that incorporates the VITA 91 high density connector in the switch slots. This specification allows double the bandwidth with speeds to 56 gigabaud per second across a single channel. This is a currently available stepping stone to the next generation VITA 100 standard, which will take some time to be fully developed and tested."
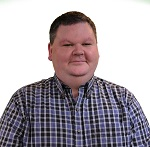
Jamie Whitney
Jamie Whitney joined the staff of Military & Aerospace Electronics and Intelligent Aerospace. He brings seven years of print newspaper experience to the aerospace and defense electronics industry.
Whitney oversees editorial content for the Intelligent Aerospace Website, as well as produce news and features for Military & Aerospace Electronics, attend industry events, produce Webcasts, oversee print production of Military & Aerospace Electronics, and expand the Intelligent Aerospace and Military & Aerospace Electronics franchises with new and innovative content.