Artificial intelligence and machine learning in signal processing become the intelligence analyst's friends
THE MIL & AERO COMMENTARY – Artificial intelligence (AI) and machine learning are poised to revolutionize embedded computing sensor processing for applications like reconnaissance and surveillance, weapons fire control, signals intelligence (SIGINT), and electronic warfare (EW).
This will be a big step forward in U.S. military capability, and moves beyond early AI and machine learning applications like image recognition, engine maintenance and prognostics, and self-driving cars.
One of today's greatest promises of AI and machine learning for military uses involves image and RF signals analysis -- not so much to determine what is significant in gathered intelligence, but to throw away the mountains of data that have no importance.
A capability like this could reduce the pressure on systems designers to provide large amounts of secure data storage and raw processing power necessary to gather and massage data that ultimately is of no interest. Think of it as using an evaporative cooler in a dry and extremely hot environment as the first stage of an air conditioning system. Instead of sending hot air from the outside environment straight to the refrigerated air conditioner, this kind of setup would send pre-cooled air to the air conditioner to improve its performance, reduce costs, and extend system life.
It could be much the same with AI-enabled digital signal processing, which could throw out irrelevant data before sending it to data-processing elements like general-purpose processors, field-programmable gate arrays (FPGAs), and general-purpose graphics processors (GPGPUs).
Systems designers and computer scientists are in the initial stages of development for these kinds of signal processing architectures that use AI and machine learning for pre-processing -- much the same as an evaporative cooler might work in a two-stage air-cooling system.
How might this work in practice? Start with the need for secure encrypted data storage for signals intelligence and reconnaissance. This kind of application today can require tens of terabytes of hard disk drive capacity just to gather sensor data. As sensors increase in sophistication, this amount of data undoubtedly will grow.
Now think of the computer power necessary simply to encrypt and decrypt this raw sensor data. No deployed military system today can operate without encryption and similar kinds of information security. This is necessary to resist the effects of enemy computer hackers and eavesdroppers.
No one can forget, furthermore, the so-called Hainan Island incident nearly two decades ago, in which the Chinese military was able to gain access to classified U.S. reconnaissance data on disk drives aboard a downed U.S. Navy EP-3E ARIES II signals intelligence aircraft that was forced to land in Chinese territory after a mid-air collision.
So think about a reconnaissance mission that over the course of several hours gathers 10 or 12 terabytes of sensor data. If this data is crucial and time-sensitive, there is a need to transmit it over tactical data links, which not only taxes battlefield communications bandwidth, but also poses an additional vulnerability to enemy hackers, spoofers, and eavesdroppers.
The alternative is processing that data aboard the intelligence-gathering platform, such as a reconnaissance aircraft. Doing this in anywhere near real time is a tall order even today for deployable data processors.
Related: AI, machine learning driving embedded bus-and-board development
But what if on-board processors didn't need to crunch all that data? Think about an airborne maritime intelligence mission. There's a lot of empty ocean to cover that offers no valuable data. The same is true for signals intelligence: there are lots of RF signals out there -- most of them, in fact -- that have no relevance. What if all that extraneous data could be tossed aside using artificial intelligence and machine learning capability?
Analysts would get important data much more quickly to translate into actionable intelligence. The intelligence-gathering platforms themselves could be smaller to accommodate on-board sensor processing ... think unmanned aerial vehicles (UAVs) vs. manned aircraft. This approach, in theory, also would have fewer demands for sophisticated computer cooling and thermal management. It's true that data processing capability is advancing rapidly today, but why brute-force it when you don't have to?
As time goes by, we'll see more and more that artificial intelligence and machine learning are the intelligence analyst's friends.
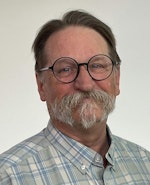
John Keller | Editor-in-Chief
John Keller is the Editor-in-Chief, Military & Aerospace Electronics Magazine--provides extensive coverage and analysis of enabling electronics and optoelectronic technologies in military, space and commercial aviation applications. John has been a member of the Military & Aerospace Electronics staff since 1989 and chief editor since 1995.