Wanted: small, efficient neural networks and machine learning for space-constrained sensor processing
ARLINGTON, Va. – U.S. military researchers are asking industry for scalable, robust, and power-efficient analog neural networks to interface with the analog outputs of conventional sensors.
Officials of the U.S. Defense Advanced Research Projects Agency (DARPA) in Arlington, Va., issued a broad agency announcement (HR001124S0022) on Wednesday for the Scalable Analog Neural-networks (ScAN) project.
The ScAN program will develop new analog neural networks that could interface directly with the analog outputs of conventional sensors and demonstrate a three-orders-of-magnitude power reduction over existing solutions.
Neural networks are part of artificial intelligence (AI) systems that teach computers to process data similarly to the human brain. It is a type of machine learning and deep learning that uses interconnected nodes that create an adaptive system that enables computers to learn from their mistakes and improve continuously.
ScAN systems will demonstrate inferencing capabilities of analog neural networks while eliminating the need for analog-to-digital converters at the raw sensor level.
Approaches like in-memory computing use nonvolatile memory in passive analog crossbar architectures, which inherently is sensitive to process, voltage, and temperature variations; parasitics; and stochastic conductance fluctuations. These approaches also are also subject to ageing and drift, and require periodic calibration or retraining to maintain performance.
Instead, the ScAN program seeks to use analog technology to increase the power efficiency of neural networks over today’s digital technology, while achieving advanced inferencing accuracy, robustness to process, voltage, temperature variations, and scalability.
Today’s neural networks typically are digital, where power consumption limits fast sensor processing, and can be too large, heavy, and power-hungry for constrained sensor applications.
Analog in-memory computing recently has been partially introduced into otherwise digital neural network systems to increase their power efficiency, but also increase the use of power-hungry A/D and D/A converters. These architectures only are reliable at small scales.
The ScAN program will demonstrate inferencing capabilities of analog neural networks while eliminating the need for A/D converters at the raw sensor level, and develop the new analog neural network processing architectures and algorithms.
ScAN has two technology areas: efficiently overcoming scaling limitations and short-term performance fluctuations; and efficiently overcoming device-dependent variations and long-term performance changes.
Related: Sensor and signal processing embedded computing at the speed of battle
The ScAN program is a 4.5-year effort that also focuses on design, simulation, and hardware development of analog neural networks. Companies chosen must use existing computing infrastructure or cloud-based services for all computing needs; no new computing hardware acquisitions are allowed.
Companies interested should submit abstracts no later than 8 July 2024, and full proposals no later than 8 Aug. 2024, to the DARPA Broad Agency Announcement Tool (BAAT) online at https://baa.darpa.mil/Public/SecurityAgreement.
Email questions or concerns to DARPA at [email protected]. More information is online at https://sam.gov/opp/c6687528fcc54113a33256ec445cd0a2/view.
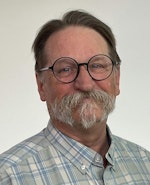
John Keller | Editor-in-Chief
John Keller is the Editor-in-Chief, Military & Aerospace Electronics Magazine--provides extensive coverage and analysis of enabling electronics and optoelectronic technologies in military, space and commercial aviation applications. John has been a member of the Military & Aerospace Electronics staff since 1989 and chief editor since 1995.